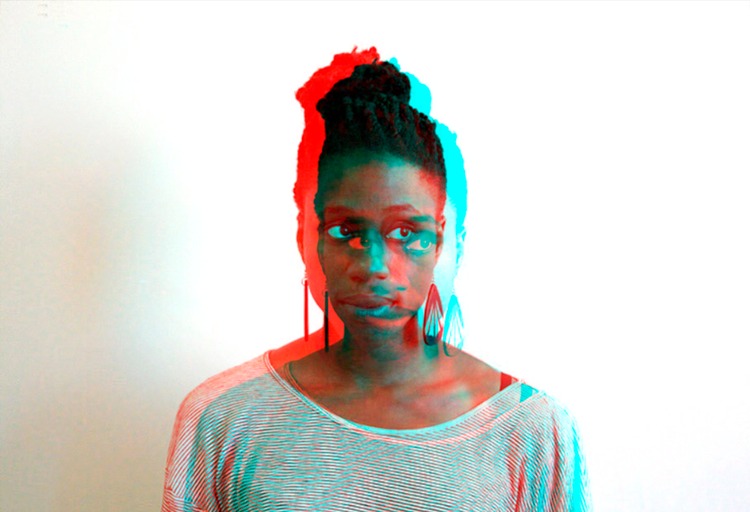
Quantifying the world
An interview with artist Mimi Ọnụọha
Mimi Ọnụọha is a Nigerian-American new media artist whose work spirals around, and deals with, the social relationships, power dynamics and other unseen forces behind data collection. The focal point of her work is the world that is being increasingly turned into data – often for reasons we know nothing about. While many of us think of data collection as impersonal statistics, Ọnụọha draws attention to the hidden motivations behind the choices and methods involved in this often seemingly dry, tedious process. She uses print, code, installation and video media to illuminate how marginalized groups are often misrepresented – or outright missed – by sociotechnical and technological systems.
Initially, her interests and studies revolved around anthropology of technology, specifically, emerging technology – “how does emerging technology change, how does it work on top of habits and customs that have existed for forever”. But after majoring in anthropology at Princeton, Ọnụọha felt that although she knew a lot about the emerging technology systems, she “didn’t have a sense of what it meant to actually create them”. Therefore she ended up in ITP – the Interactive Telecommunications Program in New York University, a graduate studies program that combines art, design and technology. Her interest and work on the methods of data collection started around 2013, following a peculiar summer she describes in this interview.
Ọnụọha is based in Brooklyn, New York. A portfolio of her work is accessible on her website.
How did you come to do what you do?
I’m a media artist, and pretty much all of my work has to do with what it means for the world to be made into data. Almost everything I do has to do with this question, and it concerns other questions like – what interrupts that process, what facilitates that process, who is affected by it, how are different people differently affected by it, what opens up when you consider what does it mean to take the world that we live in now and put it into these forms of data collection. That also explains how I ended up doing AI or machine learning, since a lot of what people think about as AI today really could refer to just machine learning. AI is just a sort of byproduct of the same process – this need to turn the world into data. We have these AI systems that make sense of these data and use it to create different predictions about the world. Just the nature of being very interested in that process of the world being made into data opens up these different gates to things like AI, machine learning, data-driven XYZ, Big Data, all of that. My educational biography combines anthropology of technology and telecommunication design, so, obviously, I have been interested in these fields for a long time. But there is another story that perhaps better shows how I got triggered into thinking specifically about the way we collect data. Would it be useful to tell it?
My educational biography combines anthropology of technology and telecommunication design, so, obviously, I have been interested in these fields for a long time.
I believe so, yes.
So really, what happened… It was probably 2013, around then. I was living in Brooklyn, and I remember… It was summer, I had been walking around the city a lot, and… I don’t know how much this happens in Latvia – it might be very specific to certain cities. I was just getting cat-called a lot. Do you know what I mean by that?
In Absentia (2019). Mixed media installation
Yes, I do. I think it happens not just in certain cities.
So I was getting cat-called a lot, and what I wanted was just a way to sort of respond to the people who were cat-calling me. But I didn’t actually want to have a conversation with anyone. I wanted to have a way to be able to interact, but not have to interact at the same time. And I thought – oh, this is actually a perfect setting for creating some kind of tech system where I could do this. I remind you, this was before Chatbox. I created the following system: if somebody cat-called me, I would go up to them and give them a piece of paper that had a phone number on it. They would then text that phone number – they could call it, but no one would answer – and I had it set up to be attached to a server, and it would send them back one of a number of randomly picked messages. So they would be having this conversation, but it wasn’t me having it – it was already pre-programed, but they had no idea. And so I did this for one summer: anytime someone cat-called me, I would hand them this piece of paper and then I would walk away. And later, when I was at home, I would log onto my computer, look at the server I set up and see what the responses were. So I did this process; it was very straightforward. It did make me feel different – I felt like I could have this conversation, but also have this distance. But then, at the end of the summer, I realized that I had inadvertently created something I had not intended: I now had this dataset with all of my cat-callers’ phone numbers. And I found this extremely interesting. This is a small semi-project; it wasn’t a real piece because I didn’t do anything with those numbers, but it did change a lot for me – it made me realize what it might mean to have this artefact, this dataset of these phone numbers that they had sort of opted into, but at the same time, had not. Kind of the same way I had and had not opted into cat-calling. I had this dataset of their phone numbers, but actually having that dataset didn’t really say anything about the process of collection that brought it into being. It didn’t say anything about this experience of having to walk up to people and give them a phone number. Nor about the strangeness I felt about it – just feeling very odd about the whole thing. That was not really captured in the end-result dataset. And that got me thinking – wow, this is something I can do so much with! But the process that brought it about is invisibilized, removed. All that matters is the artefact. I started to think – wow, there is a way that I can use this to start to think about wider systems of data collection. To think of them also in terms of a relationship between somebody who’s trying to collect something, or somebody who makes that up, and whether they intended it or not. At the end of the day, the having of the artefact goes beyond and supersedes any of the reasons for the collection. That got me going down this whole spiral – ok, what does it provide, what does it mean to have this, how do companies and different organizations approach the same process, what are the different rules in this? That one experience was really what got me obsessed with thinking about data collection as it unfolds in the world today.
In Absentia (2019). Mixed media installation
You use the phrase “turning the world into data” quite a lot. Could you perhaps untangle it? It’s a little obscure.
Yes, wonderful! I love this phrase, I use it all the time. What I’m really referring to is the whole constellation of companies, people, organizations, stakeholders and systems that are used to just collect data from the world. I’m talking about census data, about civic data as well, about how every single “like” on Facebook becomes a source of data on what you look at. All of these things. I’m talking about the fact that all these very simple acts and processes actually now generate different datasets, data points. What does it mean for that to happen? Why is that the case? It’s not that they have to do that. Why are these datasets generated, and what are they used for?
I’m talking about census data, about civic data as well, about how every single “like” on Facebook becomes a source of data on what you look at.
One example that I like that I think speaks about this very well is the Roomba vacuum cleaners. They’re round, they’re cute, they just clean your room. Wonderful. And at some point a few years ago, Roomba announced that actually those vacuums are not just cleaning your room, but, because they are connected to the Internet, they’re actually making floor plans of everybody’s apartment or everybody’s house. So the company has these massive datasets, and they said – look, we have this and we want to sell it to Apple! This is such a good example: it’s a thing that really didn’t have to happen, but it is; it’s turning some part of the world into a dataset because there’s something that can be gained from that. And I find that fascinating. In this case, it’s – ok, there’s some kind of financial advantage that can be gained. But that’s not always the case! Sometimes there’s a need to create a dataset as an intervention, as in – Look! Here’s proof of this thing that you say isn’t happening. But we’re going to collect data and show you that it is. There’s all of these different reasons for that. It all speaks of something that you want to prove, or try to make money off of, or try to make sense of. And that entire network is what I’m just very interested in.
Do you use the word “data” in the widest sense possible?
Well, yes and no. When I talk about data, I have two definitions that I use. The first one is the one coined by Australian academic Mitchell Whitelaw. He says – data are measurements extracted from the flux of the real. That’s his definition.
Why “flux”, why not just “the real”?
He means measurements that are extracted from something that changes in the world, any kind of change. So that’s his definition, and it’s taught in different universities. And I would often use that definition with my students, but I also have another, simpler, more colloquial definition that I quite like and use all the time, which goes like this: data are just things we care about and measure. Or care enough about to measure. In that sense, it’s kind of broad. But really, it comes down to quantification and the desire to quantify something. For some reason. But again, I have to say – the interesting thing is that that the reason for quantification doesn’t have to be present at the time of quantification. Yet I find it important to say that there still is a reason for it.
The Library of Missing Datasets (2016). Mixed media installation
One of your most famous works is “The Library of Missing Datasets”. Could you tell about it a little and perhaps name some of the most striking examples of missing datasets and the reasons why they are missing?
Yes, definitely. It’s a work that now consists of two different installations, as well as a research project, as well as – sometimes – a project where I work with different groups. But it started in 2015, a couple of years after I realized how interesting the methods of data collection are. I had done a few projects in the UK and came back to New York to work at the Data and Society Research Institute. And I was just very struck by the fact that there would be these spaces where lots and lots of data were being collected, and in these spaces you would see a very clear omission where there was something that just did not exist. And the thing that really got me started thinking about this was police violence. In 2015 there had just been another wave of cases of black citizens being killed by the police. At that time there was no dataset of civilians killed by the police. There was nothing like that that existed. But everybody knew that it happened, and there were lots of stories reported on it. And yet there was no dataset. However, there are lots of datasets on so many other thing related to policing, related to justice, related to crime, related to the prison industrial complex. These are huge fields not just in the U.S. literature but in universities all over the place. But there was nothing on this particular thing. And I found that just very, very fascinating. A lot of my work starts with this sort of question or hunch, just a kind of – “why?” Why is that the case? I don’t understand! So, I just started digging a bit deeper into it, and I started looking at other places where a lot of data was being collected. And again, I would find these little spots where there was nothing there. For example, in the criminal justice and incarceration system. Some of the incarceration facilities here in New York have datasets that are very clear about prisoner-on-prisoner violence, or convicted-person-on-convicted-person violence. Then there’s also data on prisoner-on-guard violence, but no data on guard-on-prisoner violence. There are these things here, and then one thing just – isn’t. Why is that? The way that I made sense of this was by actually just collecting it myself and talking to lots of people. I was asking – well, can anyone tell me, are there other examples? I was doing a lot of research, reading a lot of different things, speaking to different groups, and so I just started making these lists, these datasets, myself. The missing datasets. Things that just don’t exist, things that are omitted from being collected. At first, that was what I was focusing on – just making these datasets. But then, remember, I had already done this project in which I had seen the way in which this artefact of the dataset becomes highlighted above the reasons why it exists. And I was like – why don’t I flip this? Rather than just focusing on these datasets, let me think about why is it that certain things are not collected. I started calling these reasons for such omissions “patterns of absence”. So, what are the patterns? I came up with four, which are not exhaustive, but are the four that just seemed to fit for all the things that I was seeing. Four reasons why things are not collected.
A lot of my work starts with this sort of question or hunch, just a kind of – “why?” Why is that the case? I don’t understand! So, I just started digging a bit deeper into it, and I started looking at other places where a lot of data was being collected.
The first one: sometimes there’s an imbalance between people who have the means to collect something and those who have the incentive to collect it. Here a good example is the police cases I was talking about that have to do with justice. The data about civilians being killed by law enforcement could actually be very easily collected by law enforcement agents. In the U.S., it would be very simple to gather them and send them to one centralized place, as they do with other forms of data. So that could happen, but there is no incentive because that doesn’t look good. They don’t do it because they don’t have a reason. They have the resources, but have no incentive. On the flip side, you see people who have the incentive to collect it, but find it harder to gain resources. I think this dataset is a good example of that. Because now we do know how many U.S. civilians are killed by law enforcement agents. Now that dataset exists, but it exists because of the work of various organizers, activists, journalists, academics, and individuals acting in their own communities. Many different groups have been working together, and it has been a huge labor of these people trying to collect this. But it could have been easily collected in other ways. So that’s one reason – the mismatch between incentive and resources.
Then, the second reason is that sometimes there is a burden associated with collecting something, and that burden of collecting is not seen as being equal to what you will gain by having the dataset. For some people, it’s like – it costs a lot to collect it, but it’s worth it to have it! But for other people, it doesn’t seem that way. In those situations, a lot of examples are folks who are doing work around sexual harassment and sexual abuse. This, too, is interesting because since the time I was working on it, it seems like there has been a bit of a shift within this context – at least in the places I’ve been in. A lot of people who have experienced sexual abuse were reluctant to come forward about it because, like – if I come forward, it’s not going to solve anything; it’s going to make things harder for me. And also it means I now have to be counted within this dataset of victims or survivors… You know, there’s a cost to that. And this has changed in some ways. It’s been interesting to be doing this project for a long period of time because then you notice that a lot of it is about context and underlying conditions, not just about the attack or the data. But the question of the burden of collecting is a big one.
A lot of people who have experienced sexual abuse were reluctant to come forward about it because, like – if I come forward, it’s not going to solve anything; it’s going to make things harder for me. And also it means I now have to be counted within this dataset of victims or survivors… You know, there’s a cost to that.
The third reason is that there are just some things that are really difficult to quantify. They just resist being put into metrics. One example I often use for this is a story I did when I was working as a journalist a long time ago – a story about places that don’t show up on any of Google’s maps, and things all of these places have in common. One such place is the favelas in Brazil; then there are some lake communities in Lagos, in Nigeria, which I was particularly interested in since I’m Nigerian myself… There are some other communities that are more removed – in parts of Mongolia, in parts of Chad, etc. Places that are really difficult to reach or have very informal settlements that don’t even fit on maps. Just by the nature of where they are and how we collect this geographic data – they don’t fit, they don’t fit the systems of collection. Those systems of collection are not designed for them.
The Library of Missing Datasets (2016). Mixed media installation
But they do appear on satellite photos, right?
They always appear on satellite photos – that’s how you know they exist. They appear in astro-data. But on vector-data, the kind of maps you use when you drive somewhere – they don’t. Part of what was interesting when thinking about that story were the automated self-driving cars. We are talking about emerging technology, and it often feels like that’s removed from the things that have happened in the past. But this thing about resisting metrification – it is often very connected to historical, to past conditions. The favelas I was focusing on are real – that’s an actual city. But at that time, only 2% of the favelas were on the maps. And it’s not like it’s just the maps. People in these same favelas didn’t have their own postal addresses. There were a whole lot of state systems that they were outside of. And so it makes sense that they would also be outside of some of these digital systems that are built on top of those. So, that was the third.
The favelas I was focusing on are real – that’s an actual city. But at that time, only 2% of the favelas were on the maps. And it’s not like it’s just the maps. People in these same favelas didn’t have their own postal addresses.
A few more examples of this, maybe?
Ok. People sometimes kind of struggle to understand this one, but I was in touch with statisticians that work with the U.S. Federal Reserve, which deals with U.S. currency. And one of the things they were talking about was how they really have no sense of how much U.S. currency – cash – is outside of the U.S. Statisticians are really good in terms of talking about missing data because the main job of a statistician is to try to make sense of “missingness” by modeling. There is a whole team of folks whose job it is to try to figure out – based on the amount of money that has been printed in all this time, and based on how much we can see coming through this and this – where we think the U.S. currency (cash) outside of the U.S. is located. Where is it, and where is most of it? They do a lot of work trying to figure it out, but they really don’t know. Cash is so interesting from the perspective of data and metrification! If you compare cash transactions with credit card transactions, the latter is a perfect source of data. Everything is tracked. You can always see exactly where or to whom it goes. Cash – terrible. It’s gone in one second, you don’t know where it comes from, how much cash does one have… In so many places right now, you see this kind of switch where places are like – we don’t accept cash, only credit. It’s largely for data tracking reasons.
There is a whole team of folks whose job it is to try to figure out – based on the amount of money that has been printed in all this time, and based on how much we can see coming through this and this – where we think the U.S. currency (cash) outside of the U.S. is located.
A dollar is kind of a cultural artefact. At least in my region, many people have one-dollar bills at home just as household objects – with no intention of spending them. It’s just like – cool, I have a dollar at home – as if it was some old coin or something like that. It will probably never be used as currency.
Exactly! And how do you count that?! It’s not even just the cash that people have, that they are spending or saving – some of it is, like – I just want to have this item. So, that one is another really interesting example of resisting metrification. And it’s hard because people want cash to be counted. But the ability to know where it is and how much of it is outside the country is a hard question. So, these are the first three reasons for missing datasets.
The last one is that sometimes there is just an incentive to not have a dataset exist. And that’s a bit tricky, because I believe that every time there is a missing dataset, somebody gains by having it not exist. Even if they don’t intend to. Somebody is always gaining. However, there are some situations where people who are situationally disadvantaged, people who don’t have as much power in a certain context, are able to say – no, we don’t want this data to exist. And I have a few different examples. One that is kind of easy to understand in the context of U.S. politics is this: there is a decent number of people in the U.S. who are undocumented – they don’t have papers that would allow them to, like, simply exist legally in the country. And yet a lot of cities in the U.S. have done a thing where they’ve created these municipal ID cards. The reason they do it is partly because it confers some advantages for people who live in the city. But also, a lot of them have done it specifically for undocumented people. Because they realize that undocumented people don’t have any form of ID. And if you don’t have any form of ID, it’s hard for you to integrate into a certain system. So they created these city-wide ID cards, and by having city-wide cards, they make it so that anybody can get it – anybody within the city. You just have to prove you live in the city, but you don’t have to prove your immigration status. Many different cities have done that. New York is one of them, San Francisco is another one, and New Heaven in Connecticut also has done this. But there is a also a break between the cities that have done this. Some of these collect the information of these people and save it in a database so that they know everybody who has one of these cards. But other cities collect enough information so that they can give you a card, but then they remove the information from their files entirely. They get rid of it. And the reason they’re doing it is as an act of protection. Because they know that if they keep that information and they have this database of everyone who’s gotten the card, someone could get a hold of that database and compare it to other forms of identification and be able to tell who’s undocumented. And then, if somebody wanted to, for instance, kick out those undocumented people, they could use that dataset to do that.
There is a decent number of people in the U.S. who are undocumented – they don’t have papers that would allow them to, like, simply exist legally in the country.
In a way, municipalities protect undocumented residents from the federal government.
Exactly! Some municipalities do, not all. This is the interesting thing – in 2016, when Trump came to power, all of a sudden there was a much more conservative federal government in place, and one of the first things that happened was that those cities that kept this information were called upon to provide these databases. In New York it became an ongoing fight, where they were like – no, we don’t want to give it, but we have to, what do we do – back and forth. It ended up being fine – the information wasn’t provided; they were able to delay it and it worked out. But it is a really interesting example of this protection, where it’s like – we’re going to remove a dataset as a form of protection, because it will benefit somebody who otherwise would be negatively impacted by this.
In 2016, when Trump came to power, all of a sudden there was a much more conservative federal government in place, and one of the first things that happened was that those cities that kept this information were called upon to provide these databases.
There is an interesting gap between your work as it is displayed and the stories you tell behind it. Another, more recent work of yours is the video “The Future Is Here”, which, as far as I get it, is on the manual labor behind machine learning. How did you arrive at that?
Oh, yes! It’s all about the labor that is behind producing massive datasets. It’s mostly used for supervised machine learning, where you have to provide training data into a system and then it makes sense of that. I suppose what got me into this was a question… Well, see, I exist in a lot of spaces where people are talking about AI or machine learning, and also teaching about it. People often have this sort of view of machine learning as entirely automated. Like – wow, these computers, just look at them, they just come in, they learn, they decide, boom! And you think – yes, this is the future, it’s incredible! And I found it so, so interesting that actually, what really helps to have these machine learning systems work and have the necessary data is that people have to sit and do this very skilled and tedious labor annotating these datasets – getting them into the right form – so they can be fed into the system. And in the popular story about machine learning, that doesn’t show up at all. I just wonder – why? What does it mean to have this entire labor force completely removed? So I did a whole project in which I went on those same sites and I acted both as somebody doing the work, and also someone who hired people. But when I hired people, I just told them – look, I’m an artist, I’m doing this project, I’ll pay you, of course, but can you just send me a photo of the place where you work? Not of you – I want to know where you work. Because I’m interested in this idea – where we consider the future to be? And all the photos that came in – you can see them in this project – they are mostly from Venezuela, which made a lot of sense at the time of the project because of the political situation in Venezuela back then – a strong middle class with access to lots of computational equipment but who, at the time, needed extra income.
The Future Is Here! (2019). Video
Also Egypt came up a lot. Those were the two big places where this work was being done. What I did for the project, was – I made this video and I showed just the photos that people sent to me, but then I also had this stylized view of it where they are made into illustrations – I wanted them to be hand-drawn because I was playing with this idea of manual labor, the mechanized version, the vision of automation vs. the hand, the very tedious handwork that goes into it. I did these hand-drawn illustrations of the same spaces and made them almost like a graphic novel, and then I added on these quotes about, like, where is the future and who are the heroes. I played with that and made this video. The narrative of it is the way that this thing is talked about vs. what’s actually happening. And what is happening is far more interesting and more important, and more poignant, and more connected to longer stories. But it’s not as narrow and flat, nor sexy, as the myth of how these things are presented – how many people have a conception of it. So that’s what that work was about.
I’m interested in this idea – where we consider the future to be?
As far as I understand, the work “The Protocol of Refusal” is currently under progress?
Yes, it’s actually coming very soon.
What is it going to be about?
It is tied to this essay I wrote for a book that just came out called “Uncertain Archives”. It’s an MIT Press book to which loads of people contributed. Every contributor took a different term related to data and technology, and my term was “natural”. That was the one I chose because, again, I was interested in this idea of what we assume to be “normal” and “natural” versus how things are, so I wrote a piece about it; it’s a written piece, but I also had done a photo series that is interspersed within the piece. What that eventually is going to be is a series of images that I’ve taken in a computing center – in a server room that no longer exists. There are two kinds of threads that I’m playing with: one is this question of blackness and black people, and data about black people – I told you about this relationship of black people being able to control the process and what happens with that. I talk a lot about why it is that it is more comfortable to have people rendered in a data set that’s frozen and to be the ones in control of the terms of it. So the photo series is about that, but at the same time, the other thing that I’m playing with is that there’s (that’s also because of the question of control and who gets the terms) just a sort of quiet story that’s happening in the background of life right now. It concerns the fact that there were a lot of places that had their own server rooms; a lot of institutions – big institutions around in the 60s, 50s, 70s – would create their own server rooms and they would host a lot of their information in those rooms, and those rooms were local to them in the same city. And that has changed. A lot of those rooms are getting shut down because of big cloud computing. But cloud computing just means that you’re transfering it rather than storing it locally in your own place and having to run it yourself. You give it to Google, mostly to Amazon, and they now have their huge, huge data farms, and they store your data over there with them. And so I went – I don’t know how they let me in – I went into one of these server rooms and took the series of film photos in that server room. I’m kind of emulating black British artist Ingrid Pollard – a photographer who talked a lot about black people and where they were allowed to be in England at the time. And so I’m playing with these three things together, where it’s about this control – who gets the control, where something can be and who gets to have control over a narrative, a story, a data set. I will be showing that in an exhibition, I think, in September – you know, Covid-willing. We’ll see.
Mimi Ọnụọha